引用格式:
崔家乐, 曾祥峰, 任政威, 孙健, 汤晨, 杨万能, 宋鹏. 基于轻量化Ghost-YOLOv8和智能手机的田间水稻有效分蘖检测方法[J]. 智慧农业(中英文), 2024, 6(5): 98-107.
Citation:CUI Jiale, ZENG Xiangfeng, REN Zhengwei, SUN Jian, TANG Chen, YANG Wanneng, SONG Peng. Detection Method of Effective Tillering of Rice in Field Based on Lightweight Ghost-YOLOv8 and Smart Phone[J]. Smart Agriculture, 2024, 6(5): 98-107.
基于轻量化Ghost-YOLOv8和智能手机的田间水稻有效分蘖检测方法
崔家乐, 曾祥峰, 任政威, 孙健, 汤晨, 杨万能, 宋鹏*
(华中农业大学 作物遗传改良全国重点实验室,湖北武汉 430070,中国)
摘要:
[目的/意义]单株有效分蘖数是影响水稻产量的重要农艺性状之一,为解决水稻分蘖密集、相互遮挡且存在无效分蘖导致有效分蘖检测成本高、精度较低的问题。
[方法]通过对水稻有效分蘖与无效分蘖高度的调查分析,提出一种基于水稻分蘖高度的有效分蘖计数方法,即在水稻固定高度收割后,测量茎秆数量以得到水稻有效分蘖数;通过GhostNet对YOLOv8模型进行轻量化,以减小模型规模,便于手机端部署;并基于此模型,开发手机端水稻有效分蘖检测程序。
[结果和讨论]田间实验结果表明,在水稻植株总株高的52%~55%范围内进行收割,计数茎秆数量得到有效分蘖数,其查全率与准确率均超过99%;轻量化的Ghost-YOLOv8模型参数量减少43%;基于该模型的水稻有效分蘖App,对本研究标准下采集的100张茎秆横截面图像进行预测,准确率为99.61%,召回率为98.76%,与人工计数单株有效分蘖结果相比,决定系数为0.985 9。
[结论]满足田间水稻有效分蘖计数需求,有助于育种专家收集大量数据,为水稻产量田间预测提供基础。
关键词: 水稻有效分蘖;Android;YOLOv8;GhostNet;App;表型
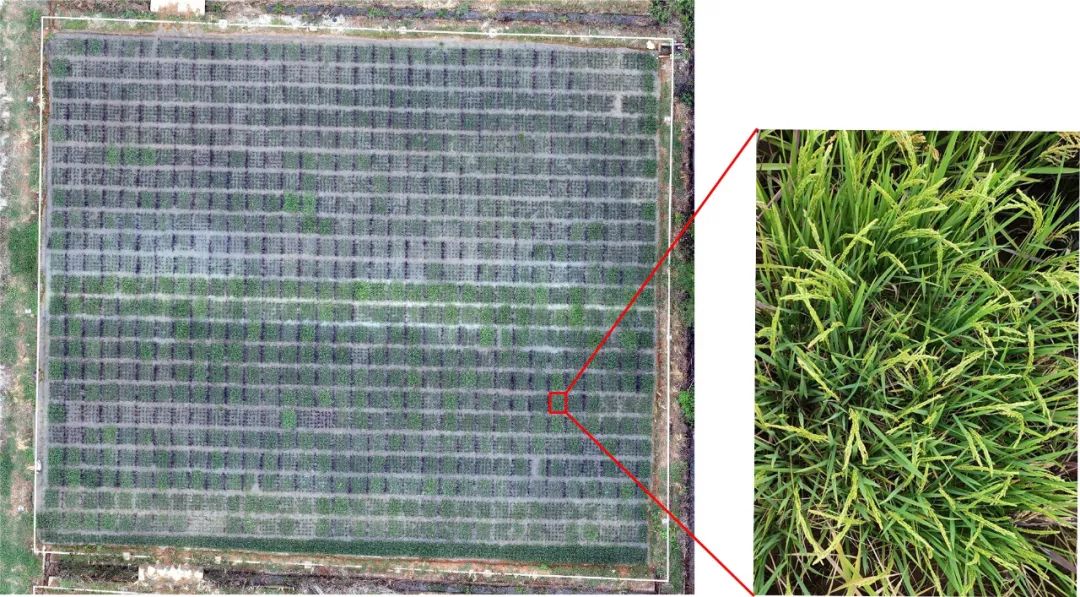
Fig.1 The rice planting scene in the experimental field of the crop phenotyping platform at Huazhong Agricultural University
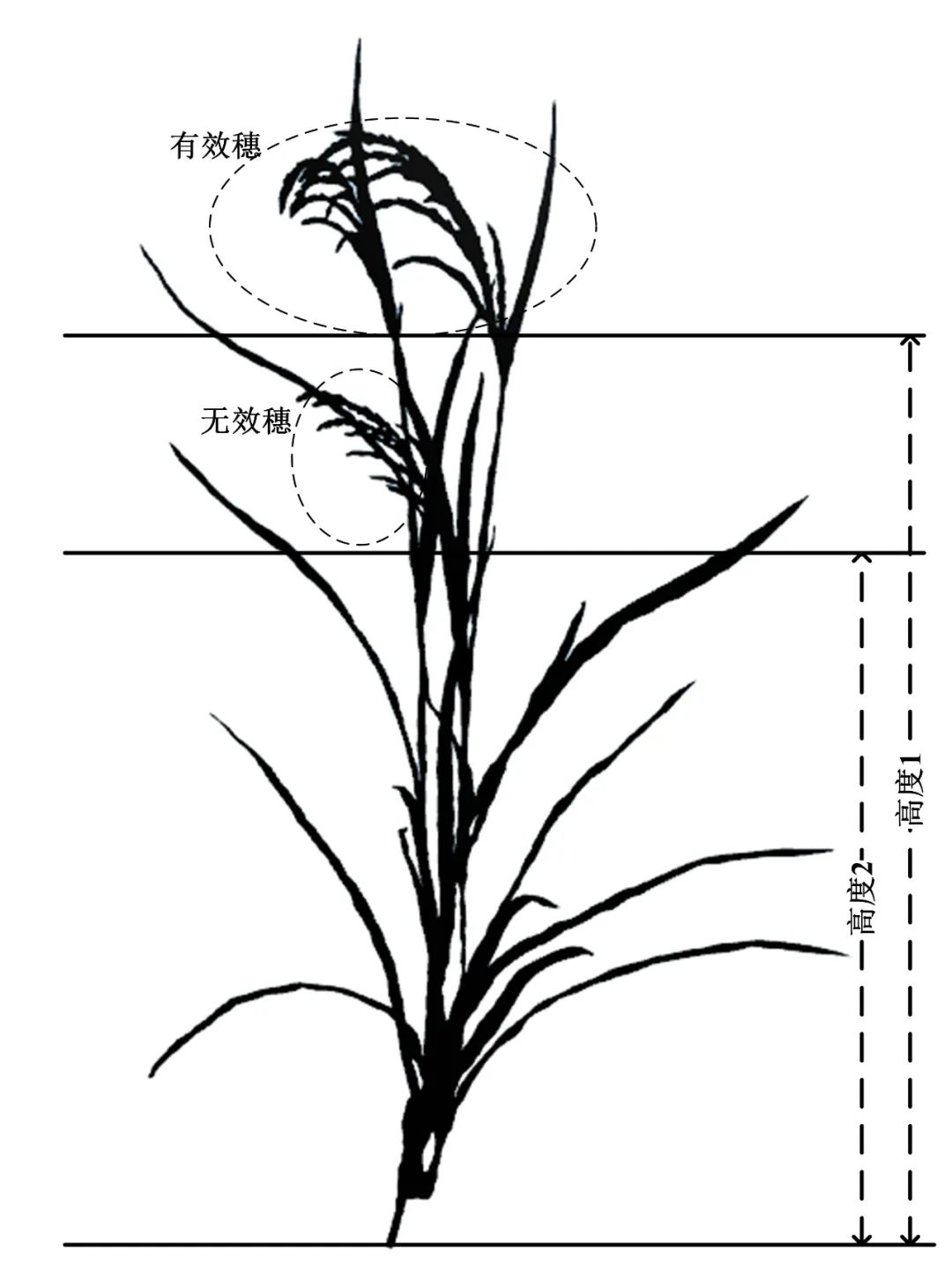
注:高度1为有效穗距地面高度;高度2为无效穗距地面高度。
图2 水稻分蘖高度调查示意图
Fig.2 Schematic diagram of rice tiller height investigation
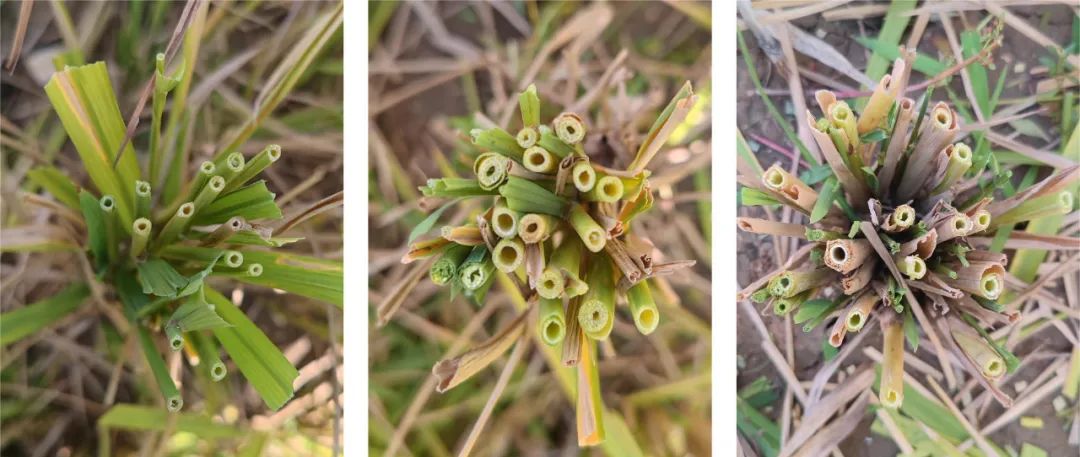
a. 高度1 b. 高度2 c. 距地面5 cm处
图3 水稻茎秆横截面图像
Fig.3 The cross-sectional image of rice stem
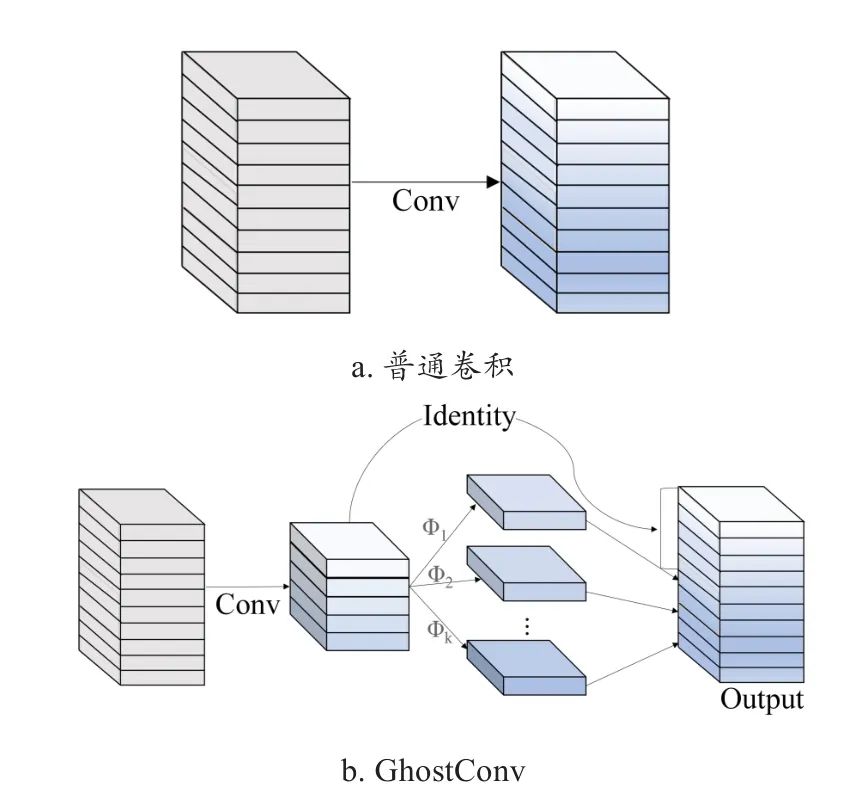
Fig.4 Ordinary convolution compared with GhostConv
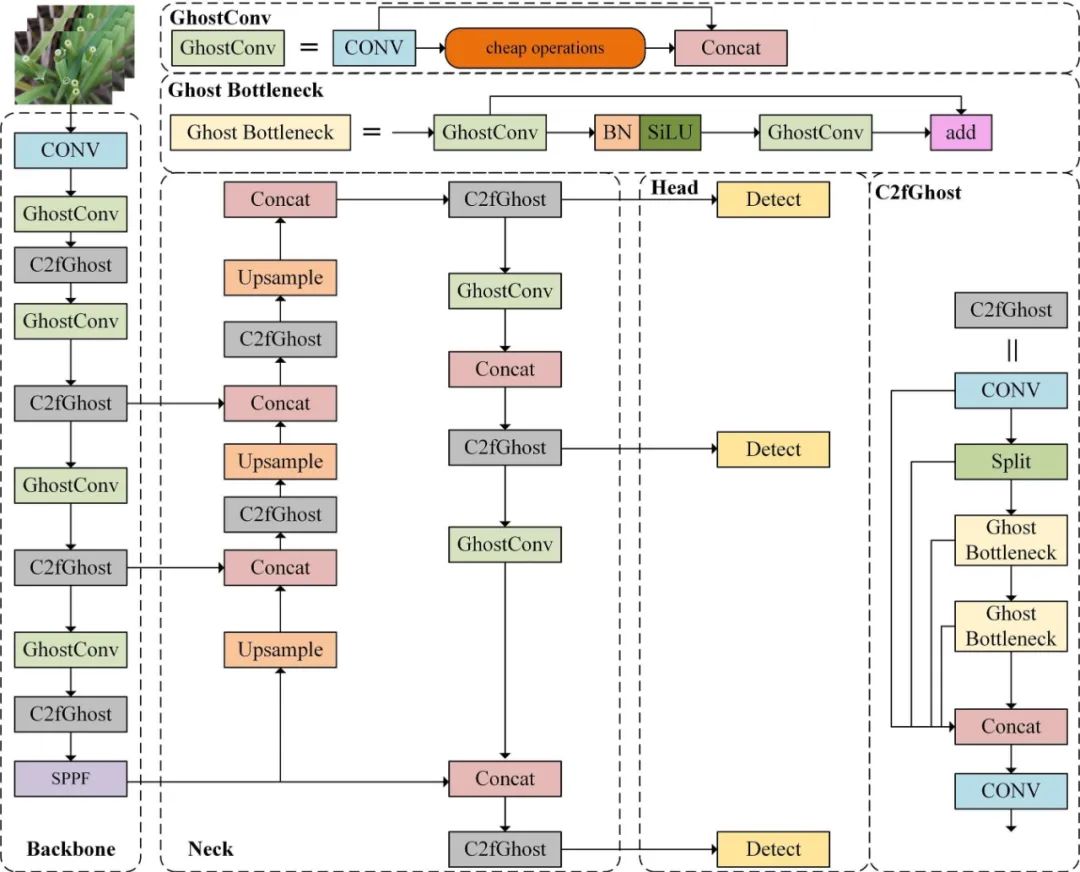
Fig.5 Structure diagram of Ghost-YOLOv8
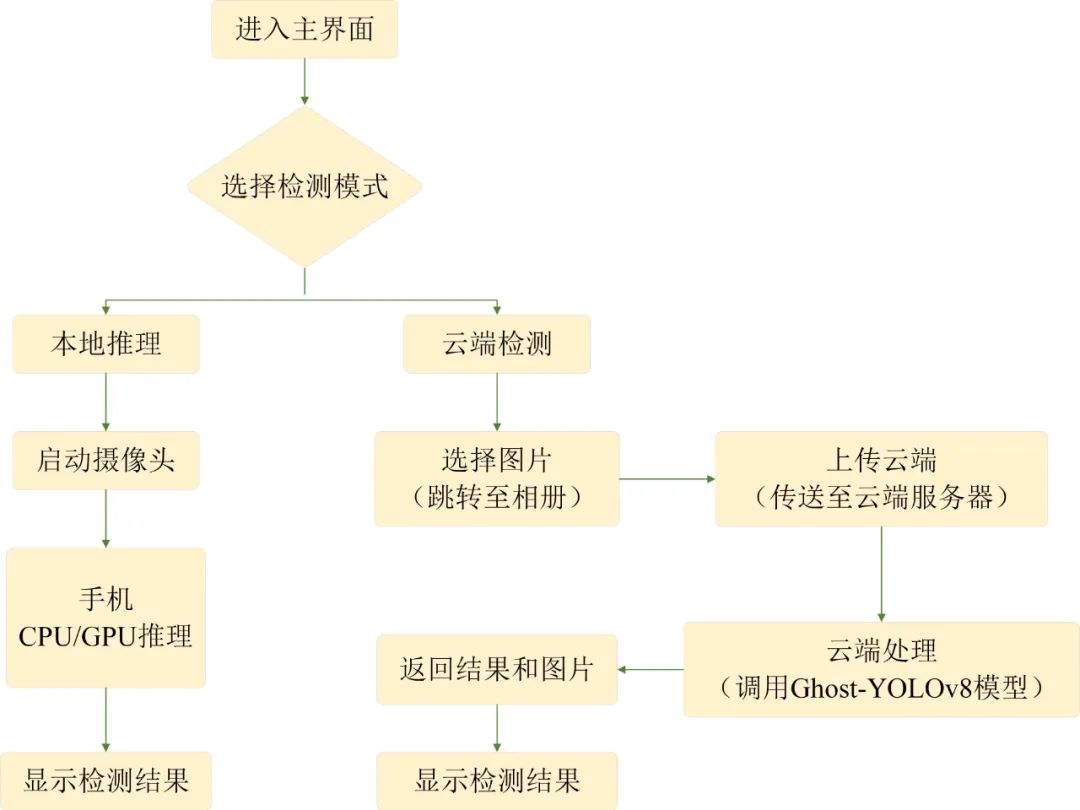
Fig.6 Workflow diagram of the rice effective tiller detection App
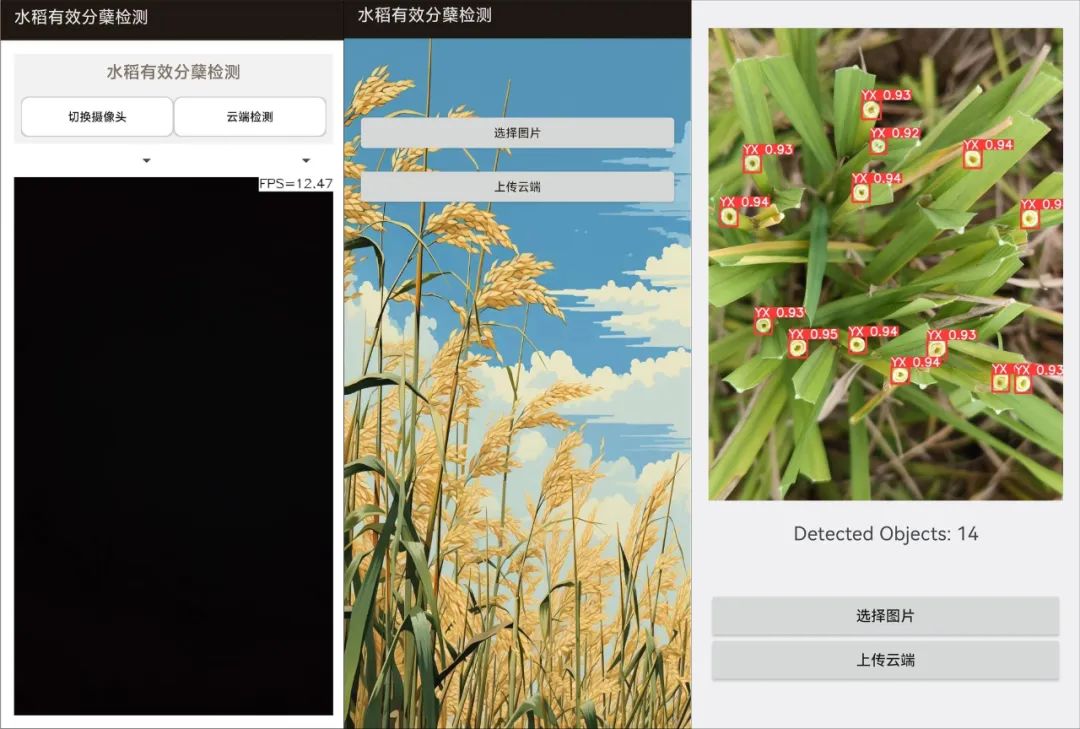
a. 主界面 b. 云端检测界面 c. 检测结果显示
图7 水稻有效分蘖检测手机App界面
Fig.7 Interface of the mobile App for rice effective tiller detection
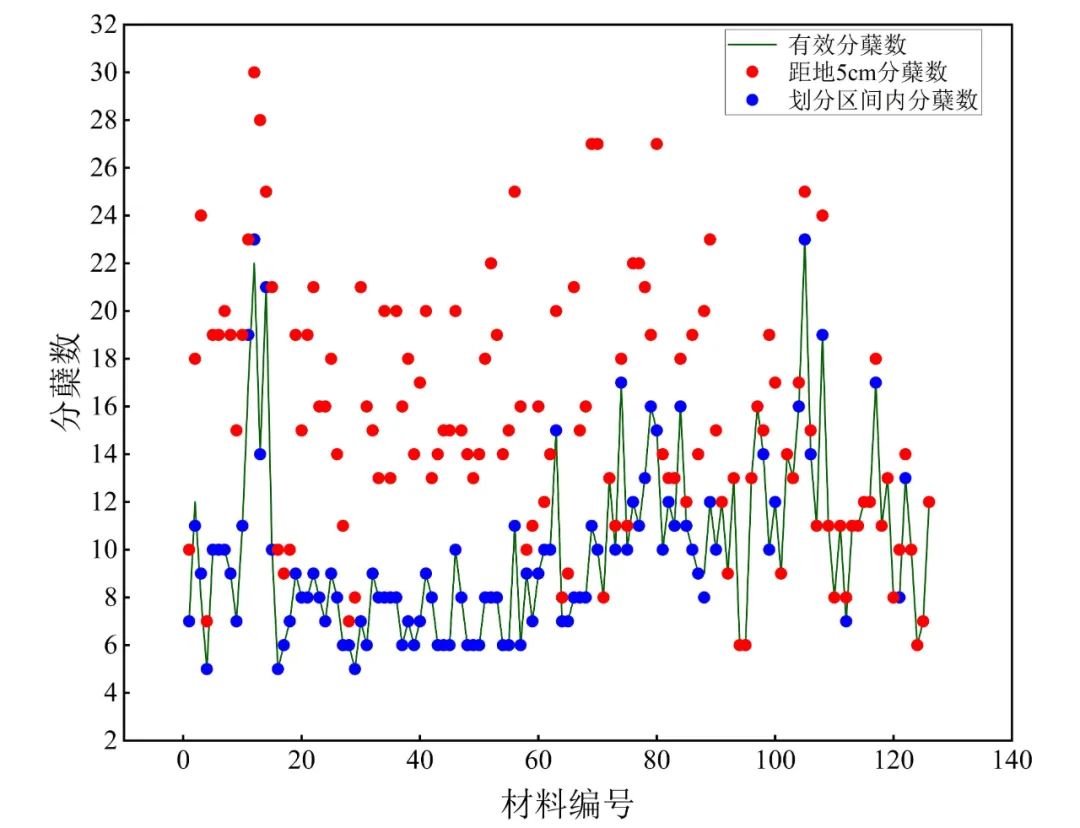
Fig.8 Comparison of tiller number and productive tiller number at different heights
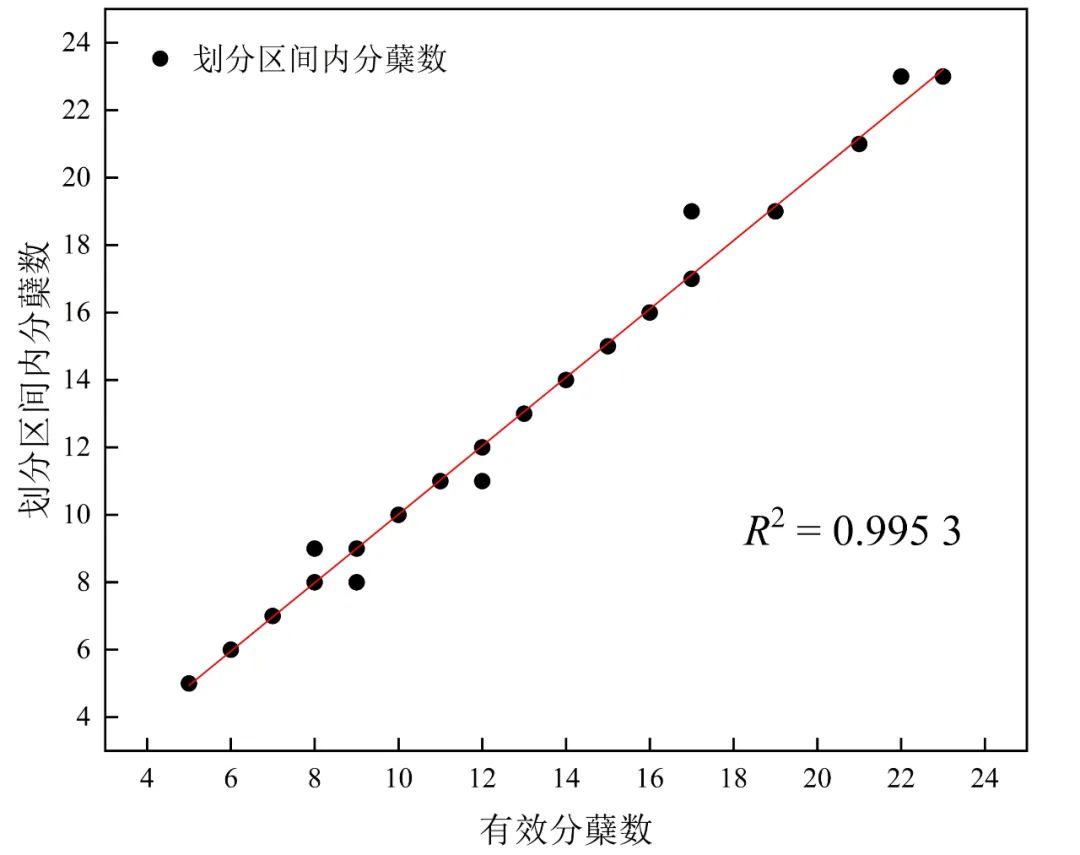
Fig.9 Comparison of the number of productive tillers in the divided interval and the number of productive tillers per plant from field surveys in 2022
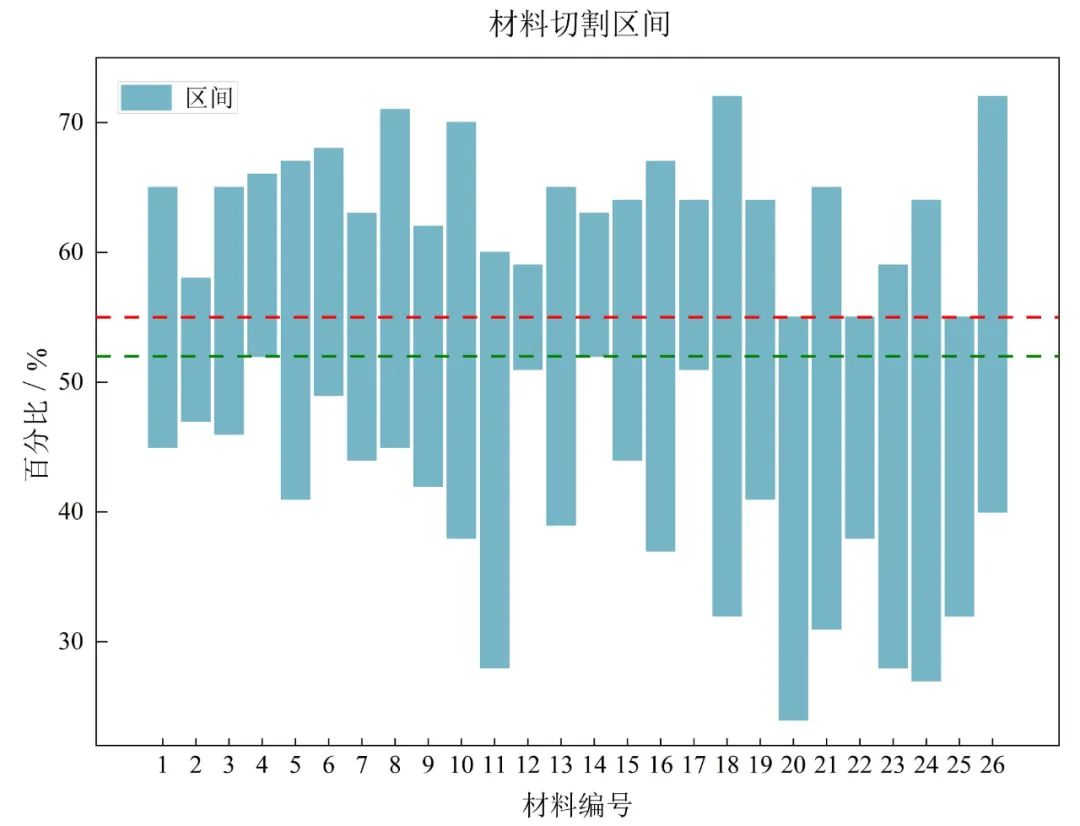
Fig.10 Delineation of regions for effective and ineffective tillers in 26 rice varieties from field surveys in 2022
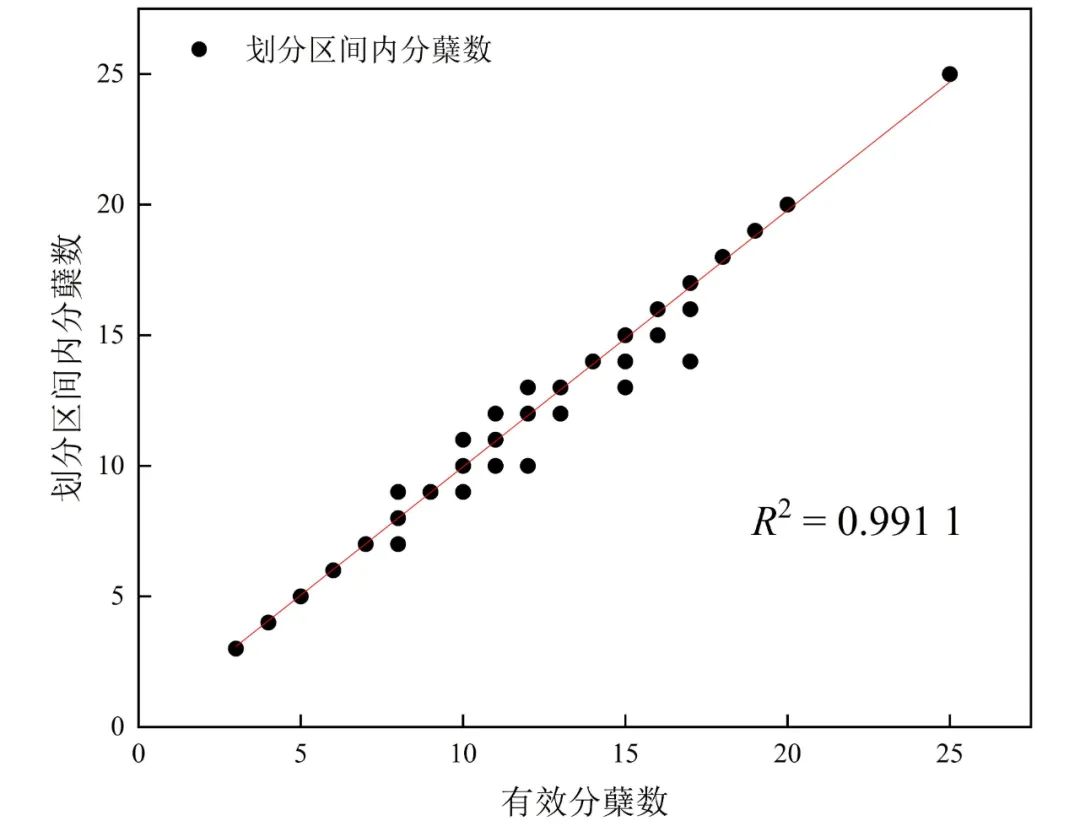
Fig.11 Comparison of the number of productive tillers and the number of productive tillers per plant from field surveys in 2023
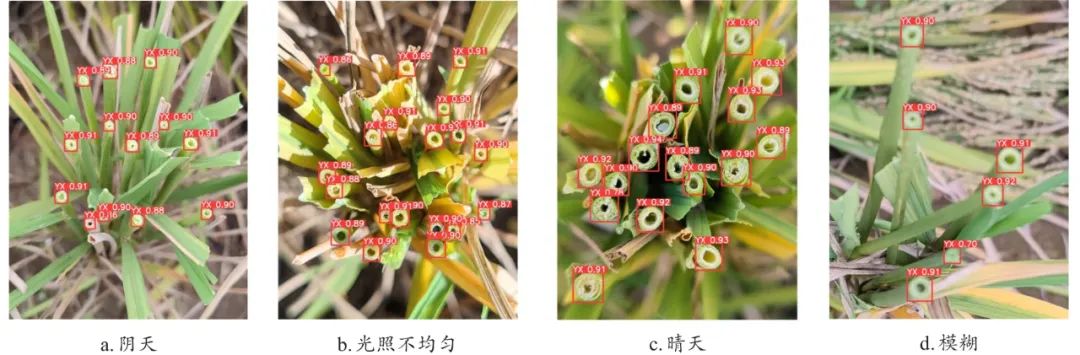
Fig.12 Prediction results of the Ghost-YOLOv8 model
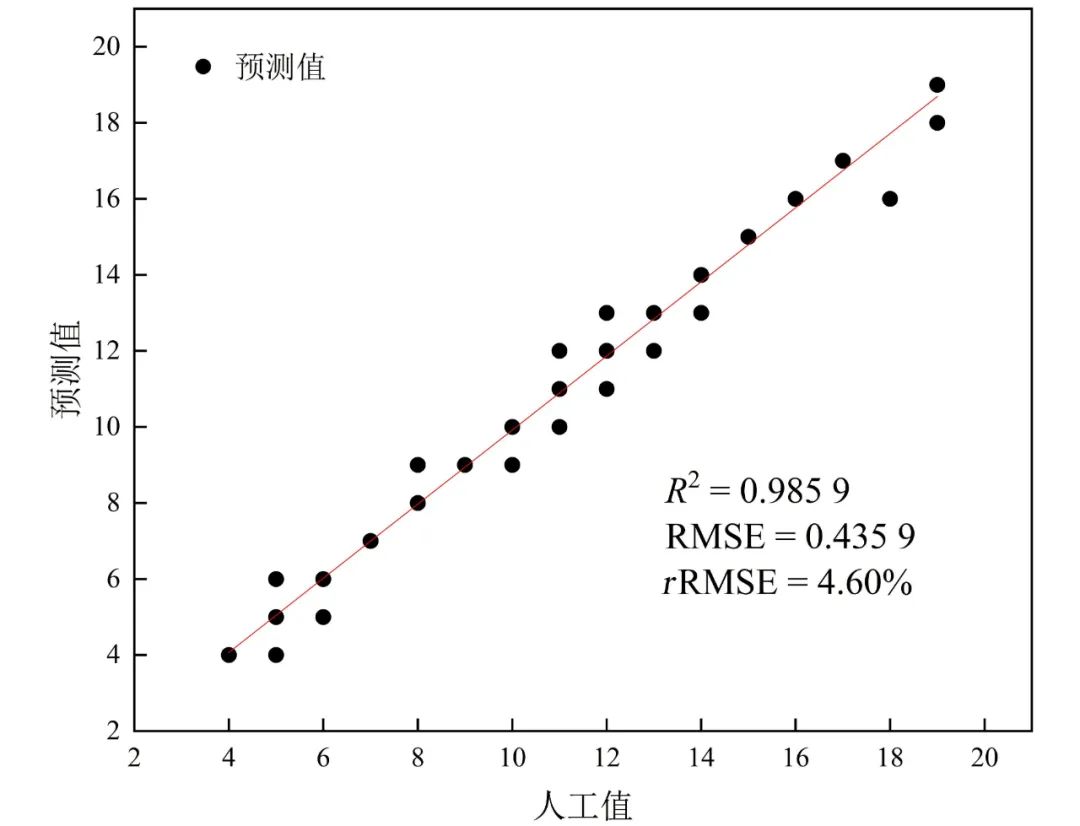
Fig.13 Linear regression comparison of prediction results from mobile App for detecting effective rice tillers versus manual results
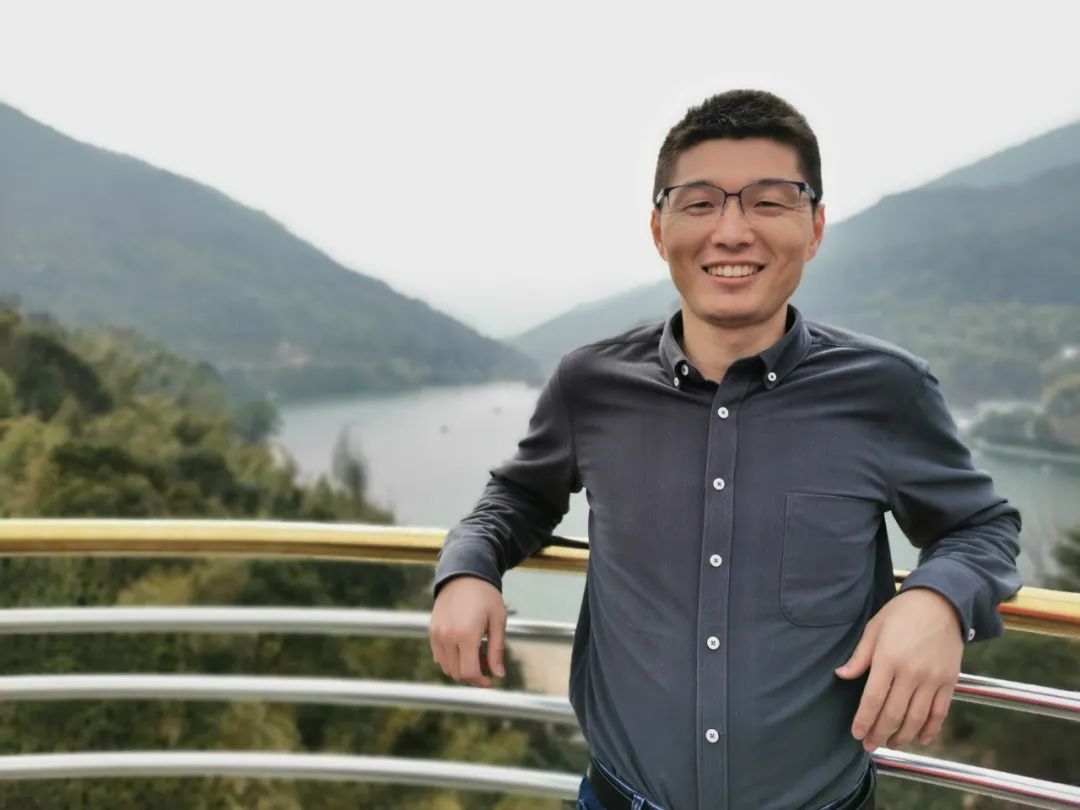
宋鹏 副教授
宋鹏,博士,华中农业大学副教授。主要从事作物表型检测方法、表型机器人技术及装备研究。《Plant Phenomics 》《智慧农业(中英文)》期刊青年编委,发表SCI/EI论文22篇,获授权发明专利24项,获湖北省技术发明二等奖、神农中华农业科技二等奖各1项,参与研发的高通量水稻表型检测技术装备获批2023年中国农业农村重大新装备,研发的表型技术在全国42家单位推广应用。
来源:《智慧农业(中英文)》2024年第5期
(转自:智慧农业期刊)
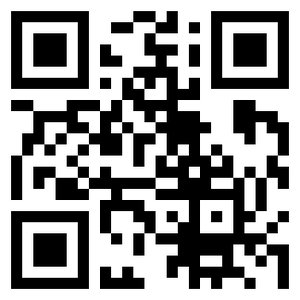
VIP课程推荐
APP专享直播
热门推荐
收起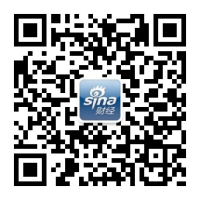
24小时滚动播报最新的财经资讯和视频,更多粉丝福利扫描二维码关注(sinafinance)