来源:钛媒体
TMTPOST -- In the fast-evolving AI landscape, the push for lower operational costs and more efficient systems is becoming crucial. At the heart of this effort lies AI infrastructure (AI Infra), a sector that is rapidly gaining importance in China’s burgeoning artificial intelligence market.
One of the most significant events in this sector occurred just before the World Artificial Intelligence Conference (WAIC) in July this year, when executives from two competing AI Infra companies took to the stage at a conference in Shanghai. Despite being competitors, both companies were focused on securing collaboration agreements with an AI chip company that could help them advance their goals.
The CEO of one of the companies, upon hearing that a competitor would also be attending, immediately reached out to the event organizers to secure an additional speaking slot. The CEO flew from Beijing to Shanghai to present the company’s vision, marking their first public address since its establishment.
This bold move paid off, as the company not only secured the collaboration with the target client but also successfully won another partnership agreement at a subsequent AI computation release event just three days later. This episode is a prime example of the fierce competition in China’s AI Infra industry, a sector that has grown to become integral to the AI ecosystem.
The Rise of AI Infra in China
AI Infra acts as a bridge between computing power and AI applications, providing critical infrastructure such as software systems, data storage and processing, and network facilities. Its goal is to address challenges such as the limitations on high-end AI computing power from U.S. firms, decoupling Chinese models from overseas GPU technology like Nvidia’s, and solving issues related to computing, storage, and communication networks.
As the demand for AI-powered applications continuously increases but China faces a severe shortage of available computing power, the AI Infra sector has emerged as an indispensable part of the country’s AI model development process.
One of the main catalysts for this growth is the strategic efforts of companies in China to reduce costs while increasing the efficiency of their AI model training and inference systems.
With the United States imposing restrictions on the export of high-performance AI chips like Nvidia’s, and the overall high cost of AI hardware, the AI Infra sector is rapidly expanding to address these issues. According to a report by CICC Securities, AI Infra is currently in its early stage but is expected to grow at a rate of more than 30% annually over the next three to five years. Meanwhile, KKR & Co. estimates that global investments in data centers could reach $250 billion annually, as demand for AI infrastructure continues to rise.
The global AI market is poised for explosive growth. According to Sequoia Capital and Bain & Company, the AI market could surge to nearly $1 trillion by 2027, with the AI hardware and services market growing at an annual rate of 40% to 55%.
Within this market, over $600 billion in investments are expected to flow into AI infrastructure. As the cost of training AI models skyrockets—sometimes reaching several billion dollars per model—there is a massive push to make AI infrastructure more cost-effective and accessible.
The Battle for Computing Power and Efficiency
Over the past 60 years, AI has revolutionized various sectors, including design, healthcare, and even the pricing of GPUs. With the new AI boom, computing power has become a critical strategic asset, a factor that determines national competitiveness.
The three pillars of AI development—chips, infrastructure, and data—are inextricably linked. Chips, such as CPUs, GPUs, and memory semiconductors, play a decisive role in determining the power of AI systems. Infrastructure, including 5G networks, data centers, cloud computing clusters, and supercomputers, drives the development of computing power. Data, in turn, is the ultimate measure of the value of computing power.
The scaling laws of AI models indicate that as the amount of computing resources and data increases, the capabilities of the models grow exponentially. Over the last decade, the growth of computing resources and data has been nothing short of remarkable.
For instance, the GPT-1 model developed by OpenAI had 170 million parameters, while GPT-4 now has over a trillion parameters—a stunning increase. The computational cost of training these models is equally staggering. For example, training GPT-3.5 on Microsoft’s Azure AI supercomputing infrastructure required roughly 3,640 petaflop-days (the equivalent of one quadrillion calculations per second over 3,640 days).
As AI models become more complex, the demand for computing power surges. The costs associated with this are also climbing. A single chip produced by TSMC's 3nm process can cost over $20,000, while a set of eight Nvidia A100 GPUs can run as high as $25 million. In early 2023, ChatGPT used nearly 30,000 high-end Nvidia GPUs to handle millions of daily requests. The cost of building the underlying infrastructure for services like GPT-powered Bing AI was estimated at over $4 billion—more than the GDP of South Sudan.
As AI models require more and more resources, the cost of training large models has become a significant barrier. For example, ByteDance’s MegaScale infrastructure, designed for training models with 175 billion parameters, requires several days of training on a large cluster, with costs exceeding millions of dollars.
Despite these high costs, the AI community faces the challenge of improving the utilization efficiency of computing power, as the training efficiency of many AI models is often less than 60%.
The Role of AI Infra in Addressing These Challenges
The role of AI Infra is becoming more critical as AI models grow in size and complexity. As Shen Dou, the executive vice president of Baidu, has noted, the explosion of AI applications and the increasing demand for model training and inference require companies to focus on improving both computing power efficiency and reducing costs.
In China, the high cost of obtaining AI computing resources and the challenges associated with China’s national strategy of channeling computing resources from the east to the west have made it even more urgent to optimize the use of computing resources. This is where AI Infra comes in: by decoupling models from hardware, AI Infra helps ensure that computing resources are used more efficiently and that AI models can scale more rapidly without incurring prohibitive costs.
Companies like SiliconFlow and Infinigence have introduced innovative AI Infra solutions. Infinigence, for example, has achieved a 90% increase in computational efficiency for one of its internet clients, significantly lowering the cost of running AI models. AI Infra solutions will allow companies to achieve scalable profits within the next three to five years, Xia Lixue, the co-founder and CEO of Infinigence, told AsianFin.
The Commercialization of AI Infra
Rapid progress is being made in the commercialization of AI Infra. Startups in this field are offering a range of solutions to address the growing demand for efficient AI model training and inference. These solutions include cloud-based AI platforms, software-as-a-service (SaaS) offerings, and end-to-end solutions that combine chips and software. Companies like SiliconFlow and Infinigence are leading the way in providing customized computing power solutions to meet the needs of developers and businesses. As AI models continue to grow in scale and complexity, the demand for efficient and cost-effective infrastructure solutions will only increase.
In contrast to established tech giants like Nvidia, which dominates the global AI infrastructure space with its A100 and H100 GPUs, China’s AI Infra companies are still in the early stages of development. However, they are catching up, and their ability to tailor solutions to local needs positions them as key players in the domestic AI landscape.
Nvidia CEO Jensen Huang recently admitted that since the birth of general-purpose computing 60 years ago, there has been a shift toward accelerated computing. Through parallel computing, GPU-based computing power has significantly surpassed that of CPUs.
The development of neural networks and deep learning has also accelerated the speed at which computers acquire knowledge, bringing about a leap in computer intelligence. He believes that traditional computing methods rely on pre-set algorithmic models, lacking the ability to learn and understand. However, by incorporating deep learning, systems can adjust and optimize data to enhance the utilization of computing power.
Huang emphasized that computing technology advances a million times every decade, and within just two years, Nvidia and the entire industry will undergo profound changes. He described the future of AI as "incredible," believing that AI has narrowed the technological gap between humans, and in the next ten years, computing power will increase another million times.
"I am increasingly confident that if China wants to develop its own ecosystem and its own AI, it must have full control over the entire industry chain. After accumulating experience in optimizing AI hardware and software, we will push China to fully utilize its computing power in the age of large AI models and compete with the United States," said Professor Wang Yu, founder of Infinigence, department head of Tsinghua University Department of Electronic Engineering, in Mandarin and as translated by AsianFin.
As the competition heats up, the ability to reduce costs and enhance computational efficiency will be pivotal for AI Infra companies striving to support China’s rapidly expanding AI model ecosystem.
With a projected global investment in AI infrastructure of over $600 billion by 2027, the stakes are high. Companies that can deliver innovative, cost-effective solutions will have a significant role to play in the AI revolution.
特别声明:以上内容仅代表作者本人的观点或立场,不代表新浪财经头条的观点或立场。如因作品内容、版权或其他问题需要与新浪财经头条联系的,请于上述内容发布后的30天内进行。
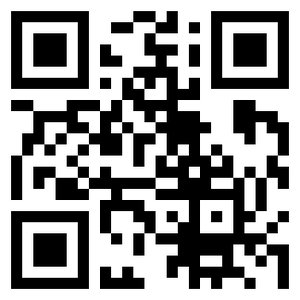
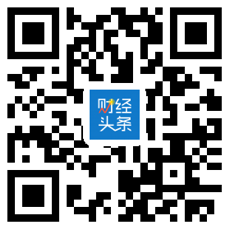
400-690-0000 欢迎批评指正
All Rights Reserved 新浪公司 版权所有